
GreenNLP
Controlling the Carbon Footprint in Sustainable Language Technology
Language technology plays a central role in the ongoing process of digitization. IT companies invest resources in the development of language models and downstream applications that process large quantities of language data with an unprecedented quality. However, deep learning, that is behind the recent success, is heavy in terms of computation and the requirements are growing dramatically. The recent developments have resulted in models that are challenging to deploy on standard hardware and whose environmental impact is of growing concern.
The GreenNLP project will address this by applying and further developing techniques of resource optimization, knowledge distillation and multilingual transfer in order to create compact solutions that reduce hardware requirements while at the same time serving more languages with the same model. The project will address two major building blocks in modern natural language processing (NLP): pre-trained neural language models and neural sequence-to-sequence translation models.
The focus is on advancing techniques that produce models with a small environmental footprint. In addition to the methodology and the models, the aim is to openly document and freely distribute all knowledge needed to successfully train the NLP models. This will help to foster the reuse of existing resources, decrease waste of computation time, and disseminate the information needed to develop NLP in a manner that is mindful of the environment and which contributes to the green transition of sustainable NLP.
The GreenNLP project consortium is formed by the language technology groups of the University of Helsinki and the University of Turku, and CSC – IT Center for Science. The two research groups represent the vast majority of NLP research in Finland. The NLP expertise of the research groups is complemented by expertise in hardware and algorithmic optimization brought by the primary computational infrastructure provider in Finland, CSC.
CSC will lead the GreenNLP work package on reducing computation with efficient training procedures, which addresses the practical optimisation of deep learning in the environment of high-performance computing (HPC). The aim is to develop a set of general guidelines and recommendations that can be applied in different HPC environments in order to optimally make use of the compute nodes and reduce the waste of resources. CSC will also contribute to decreasing runtime costs through the development of compact language models and compact translation models. CSC will additionally lead the work package on reuse and sustainability, in which the overall goal is to make as many models as possible available and to motivate the NLP community to reuse, adapt and provide models as widely as possible.
This project has received funding from the Research Council of Finland under funding decision No 353166.
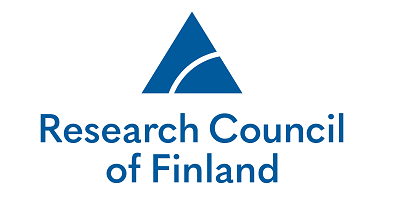
Rahoittaja
Research Council of Finland