
CryoSCOPE
CRYOsphere Science Concluding in new Observations and Productive Exploitation
The CryoSCOPE project addresses challenges related to evolving land ice, snow, and permafrost emphasising the connection to water sources and fluxes. The objective is to understand and quantify physical and chemical processes of the Cryosphere-Atmosphere-Hydrosphere (CAH) system in diverse landscapes. This encompasses examining how these interactions are represented within regional process-based models and global Earth System Models (ESMs) including AI based emulators, and predicting their susceptibility to climate change impacts.
CryoSCOPE aims to demonstrate excellence by:
- Providing advanced observations of the CAH system in globally significant ”cold spots,” including the Indian Himalayas, the Alps, Iceland, Northern Scandinavia, and Svalbard.
- Integrating observation-constrained modeling, spanning from detailed process models to ESMs, enhancing our understanding of physical and chemical processes of the CAH system.
- Generating new knowledge on cryosphere-ecosystem changes in targeted regions and globally, enabling predictions of the impacts of climate change and related drivers across seasonal to long-term time scales.
- Developing societally impactful services and solutions to bolster resilience against the changes.
As part of the project consortium, CSC is contributing to setting up the synthetic coupled process model of glacier dynamics and developing the robust hydrological model for hydrological processes in a coupled glacier-permafrost system in Svalbard. CSC is leading the task for producing a representative simulation model of present-day glaciation and permafrost distribution as well as thermal spin-up of the glacier. CSC will participate in testing the synthetic coupled process model of glacier dynamics for realistic case studies using real-world data.
CSC is additionally leading the task on coupled process Elmer/Ice simulations and emulator training for glacier lake outburst floods (GLOFs). CSC is also involved in GLOF hazard mapping, which will utilise both the process-based model (Elmer/Ice) as well as the machine-learning-based model IGM to construct local and region-wide hazard maps that can be used for effective risk-assessment.
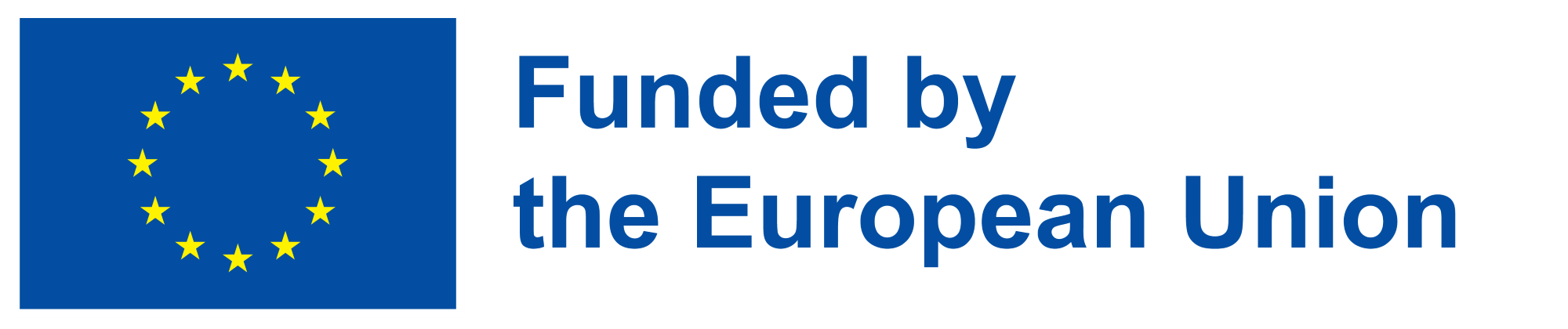